Hello guys, today we will learn what is deep learning and difference between Machine Learning and Deep Learning and also a small introduction about Neural Networks.
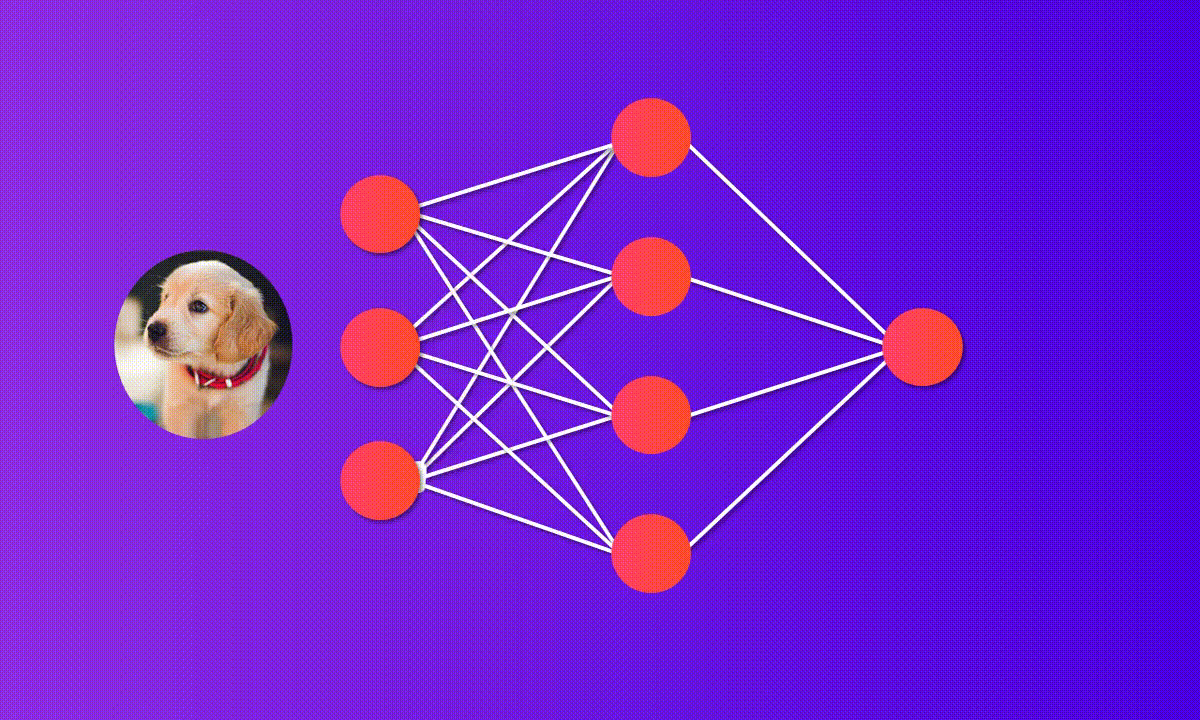


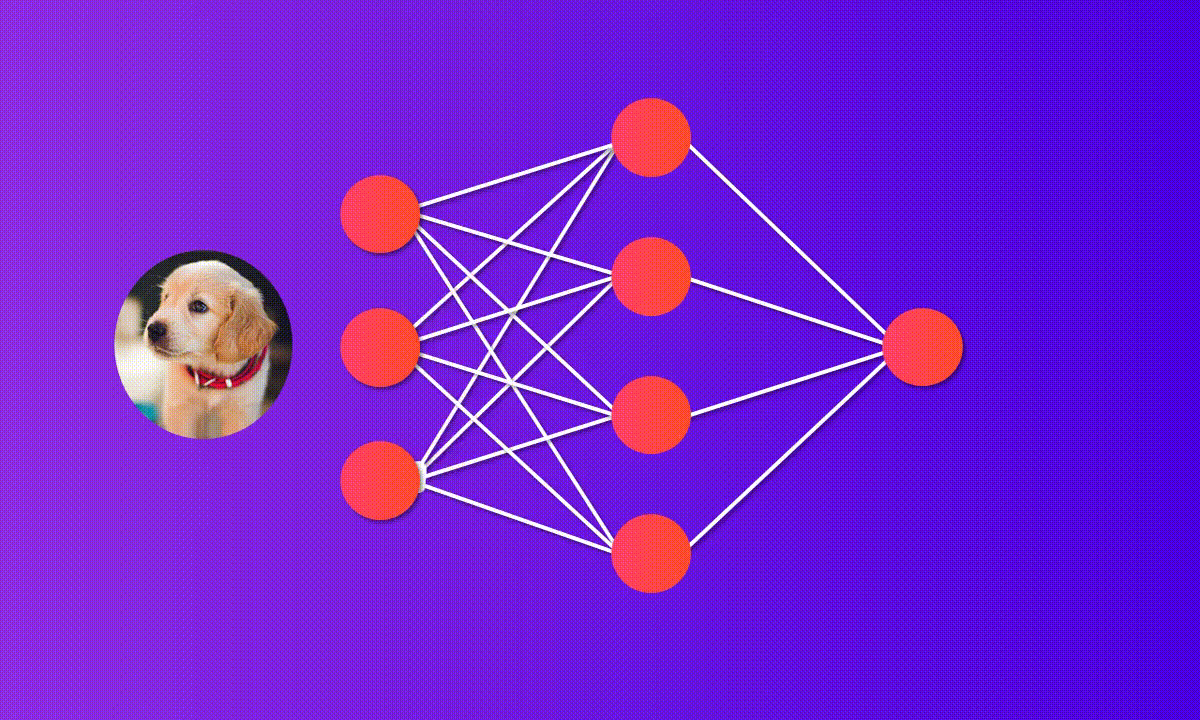
What is Deep Learning?
Deep learning is a type of machine learning (ML) and artificial intelligence (AI) that imitates the way humans gain certain types of knowledge. Deep learning is an important element of data science, which includes statistics and predictive modeling.
Difference between ML and DL

In machine learning, first we should extract features from input and then fed to the model. But when comes to Deep learning there will be no need of feature extraction. There is one of the most important algorithms called Neural Networks which we will discuss in this post.
Lets discuss some more concept about the difference between ML and DL. Before that lets know about different types of data. There are two types of data
- Structured Data - Price of house, user ID etc.
- Unstructured Data - Images, audio, text, etc.
Now, for ML models we can directly give input if it is a structured data. But when comes to unstructured data first we have to extract the features(pixel values for images, audio frequency for audio, etc.) and then fed to the ML model. Now, Deep Learning comes in the picture. Artificial Neural Networks itself extracts the features and fed as input to the model.
The above figure also show one of the main differences between ML and DL.
Artificial Neural Network
Artificial neural networks are popular machine learning techniques that simulate the mechanism of learning in biological organisms. The human nervous system contains cells, which
are referred to as neurons. The neurons are connected to one another with the use of axons and dendrites, and the connecting regions between axons and dendrites are referred to
as synapses.
There are 3 different types of ANN(Artificial Neural Networks)
- Deep/Fully Connected Neural Network
- Convolutional Neural Network
- Recurrent Neural Network
That's all for this post. From the next post I'm going to start projects on Deep Learning. You can see my Introduction to Machine Learning post here
Thank you!
Contacts:
ph.No: +91 9182530027
gmail: hunnurjirao2000@gmail.com
github: github.com/hunnurjirao
Comments
Post a Comment
If you have any doubts please leave it in a comment box